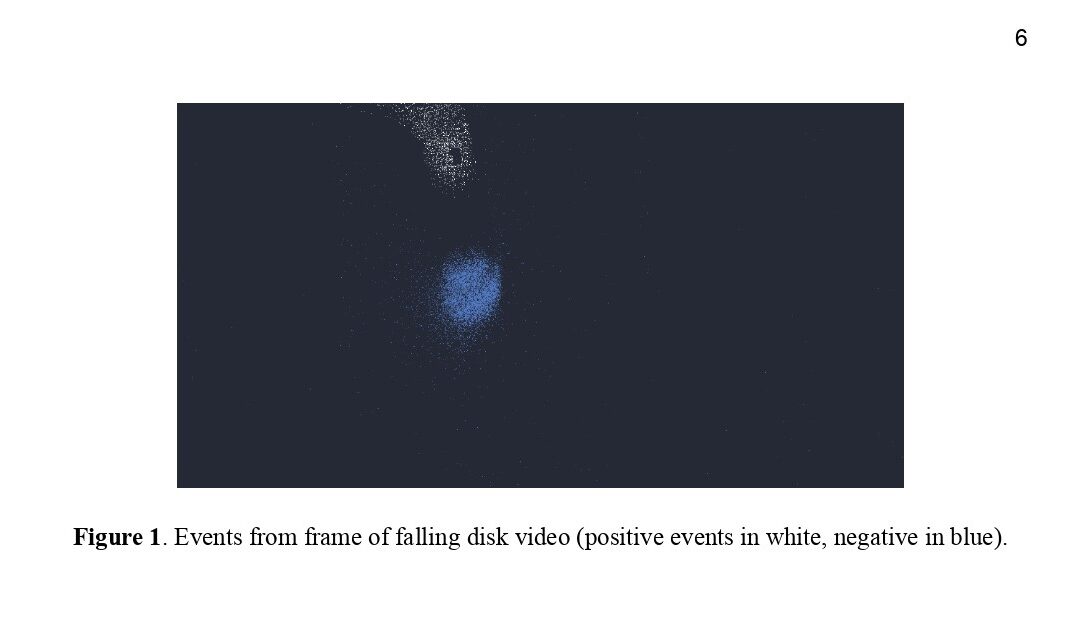
Object Tracking with an Event Camera
This paper analyzes the synth-to-real domain shift in event data, i.e., the gap arising between simulated events obtained from synthetic renderings and those captured with a real camera on real images.
This paper analyzes the synth-to-real domain shift in event data, i.e., the gap arising between simulated events obtained from synthetic renderings and those captured with a real camera on real images.
This work builds an event-based SL system that consists of a laser point projector and an event camera, and devises a spatial-temporal coding strategy that realizes depth encoding in dual domains through a single shot.
Depuis quelques années, l’utilisation des caméras événementielles est en plein essor en vision par ordinateur et en robotique, et ces capteurs sont à l’origine d’un nombre croissant de projets de recherche portant, par exemple, sur
le véhicule autonome.
This paper proposes an object detection method with SNN based on Dynamic Threshold Leaky Integrate-and-Fire (DT-LIF) neuron and Single Shot multibox Detector (SSD). First, a DT-LIF neuron is designed, which can dynamically
adjust the threshold of neuron according to the cumulative membrane potential to drive spike activity of the
deep network and imporve the inferance speed.
This paper focuses on event-based visual odometry (VO). While existing event-driven VO pipelines have adopted continuous-time representations to asynchronously process event data, they either assume a known map, restrict the camera to planar trajectories, or integrate other sensors into the system. Towards map-free event-only monocular VO in SE(3), we propose an asynchronous structure-from-motion optimisation back-end.